In today’s world, marketing is more important than ever before. It is more than just the key element behind many campaign triumphs. It also plays a crucial role in determining which technologies, goods, and viewpoints merit our time.
The relevance of marketing on cultural, economic, and technological change has elevated what was formerly seen as a trade or art to a science.
Today’s marketing experts must be well-versed in human psychology, politics, sociology, and other disciplines. Data, on the other hand, remains one of the driving forces in the industry, and in many cases integrating all of these elements is difficult.
Harvesting relevant data from human behavior is an important goal in marketing. To achieve this, numerous new approaches and techniques have emerged throughout the years. One of the most common methods is A/B testing.
What makes A/B testing such a powerful tool for marketing experts? How does A/B testing work? What are some of the most popular A/B testing tools available out there? How can you use A/B testing to quantify human behavior and improve the success of your campaigns?
In this article, we will try to give an answer to all these questions, hopefully providing you with a refresher (or lesson) on why you should be using A/B testing as a means to improve your marketing efforts.
What is A/B testing?
Before talking about how A/B testing works, we need to define what it is. At its core, A/B testing is a method by which we compare two variables to find out which one performs better.
When you see someone shopping and asking other people about which shirt looks better… That’s A/B testing at the most casual level. But for our purposes, we’re talking about the application of A/B testing in marketing experiments.
Marketing experts use the same concept to find out how people react to their campaigns, allowing them to improve them in the process.
In a nutshell, A/B testing is a form of statistical hypothesis testing that has been adapted from hard sciences to provide a dependable method to compare outcomes.
How does A/B testing work?
While the concept of A/B testing is pretty simple and easy to understand, there are several elements that need to be considered to ensure not only its efficacy but also its validity.
After all, A/B testing is a scientific method technique that is being applied. As such, it is susceptible to contamination and other factors that might compromise its results.
For the remainder of this article however, we will be focusing on the application of A/B testing to marketing. More specifically, to the testing of landing pages.
The first step when running an A/B test is to decide which variable you want to have tested. This variable might vary depending on your landing page, the objective of your campaign, approach, etc. However, it can be anything from a button’s location or color to a certain offer included on the landing page.
Once the variable has been identified, an objective is determined. When using A/B testing on a landing page, the objective will most likely be its conversion rate, although there could be other data you are interested in depending on specific cases.
Now, having determined the variables to test and the objective of the test itself, the next step is running the test itself. As we said, the test consists of showing the two (or more) versions of the variables to real users and checking if the objective was fulfilled or not. Did a conversion action occur? Which version was the winner?
After running the test with enough users, a pattern will start to emerge. Version A or B of your landing page will show a higher success when it comes to converting users. Once this difference in the conversion rate reaches statistical significance (or doesn’t after a long time), the experiment can be stopped.
You can now say which version is more effective at converting visitors into customers… well, kind of.
As we said before, there are several variables that can influence the results of an A/B test. This takes us to another question: What variables should be considered when running an A/B test to ensure its validity?
The caveats of A/B testing
You might be thinking “Well, that didn’t fully explain how does A/B testing work…”, and you would be right. While in the previous section we described the basic steps involved in A/B testing, there is so much more to it than just looking at the steps.
If you paid attention, we said that an A/B testing experiment would end when statistical significance was reached or it wasn’t reached after an extended period of time. Well, this is an essential concept that needs to be highlighted.
People are not as easy to understand as psychology, statistics, and marketing books would have you believe. While everybody knows this fact, it is not unusual for marketing experts to forget about it when testing. There are a ton of factors that can influence how someone behaves during your A/B testing.
What was your visitor’s mood? Were they using a mobile device? Were they in a hurry? Have they recently acquired a similar product? They are a potentially infinite number of elements that could affect the decision beyond the different versions of the element you were evaluating.
Here is where one of the elements you need to consider when talking about statistical significance comes into play: Randomization.
The different versions of your element should be presented to users in an entirely random way. Randomization decreases the chances of “contamination”, which would be if other factors influenced the outcome. Remember, you want to test “A” and “B”, not a “C” you can’t determine or account for.
While A/B testing is one of the most basic forms of randomized control experiments, lacking placebo groups and similar elements, it still requires researchers to keep several things in mind. Randomization is essential but it shouldn’t be applied lightly.
For example, if you are trying to identify which version of a landing page is more effective at converting visitors using a mobile device, you should limit the A/B test to mobile devices. The randomization should be limited to the sample, a concept known as blocking.
Another difficulty that arises with A/B testing, is that you usually won’t be testing “A” vs “B” as independent elements but as a set composed of determined or interchangeable elements. As you can see, this increases the complexity of running A/B tests. After all, it is not effective to test on a 1v1 basis.
All of these factors, which are only a few of the many that should be considered, make A/B testing more complex than just showing “A” and “B,” and taking notes along the way. That’s without taking into account the interpretation of the results which can be more complicated than designing the test itself.
How to make A/B testing easy?
“Yes, I get it… A/B testing is hard”. If you are thinking this, welcome to the club. At some point, most marketing experts found themselves scratching their heads… if they actually tried to understand the science behind A/B testing.
It is not a surprise that advanced statistics was a requirement to take part in the world of marketing. Fortunately (or unfortunately if you like crunching numbers), this has changed over the past years. Sure, it is still extremely valuable to have an understanding of how to interpret A/B testing results, but technology has made it easier than ever to run A/B tests.
Don’t misunderstand. It is still essential that you understand how A/B testing works. You can’t design the experiment without understanding this, after all. However, when it comes to interpreting and running the experiment, you can just let a machine take care of it.
One of the most problematic aspects of online A/B testing in the past was the lack of automation. This meant that implementation costs were pretty high. Now, swapping between variables back and forth is extremely easy and cost-efficient.
This is possible due to platforms like Google Optimize, VWO, ABtesting.ai, and more. These solutions made A/B testing highly accessible to the public while also providing powerful features like targeting tools, advanced statistical modeling, multi-armed bandit testing, multivariable testing, heatmaps, visitor recordings, and more.
All of these features have made these platforms an essential tool in the toolbox of every marketing specialist worth their salt. After all, A/B testing is a reflection of a search for constant improvement, which should be at the heart of individuals and businesses, especially at a time when customers’ needs and perceptions change at an unprecedented rate.
How to use A/B testing to improve your campaigns?
Using a software solution like Google Optimize, VWO, or ABtesting.ai is not enough to improve your marketing campaigns. While these tools will certainly help, you will only notice their effect if you use them correctly.
As such, there are several common mistakes you want to avoid when doing A/B testing. The biggest (and most common) of these mistakes is not letting the test reach statistical significance. This usually happens due to impatience by the people running the test or their client as they want to implement changes as soon as possible to maximize conversions.
Unfortunately, implementing a change without letting the test finish can have the opposite result. By achieving statistical significance you will ensure that the result was not just a coincidence or derived from “noise”, which will, in turn, improve the result of your test.
Another common mistake is trying to consider too many variables at the same time. While today’s solutions can test multiple variables at the same time. The more variables or metrics you are considering, the harder it will be to determine what is working from what is not. Consider that even a failed experiment (if you don’t get a “lift”) will provide you with valuable information.
Finally, don’t sleep on your laurels. While a well-done test will undoubtedly provide you with relevant and practical data, retesting is an often wise move.
This will not only allow you to continue improving your conversion rate but also ensure you were not the victim of a false positive error. If you get contradictory information, test again! Is better to find out early than doing so once you have caused irreparable damage.
As you can see (and probably already knew), A/B testing is a powerful tool but a tool nonetheless. Now that you—hopefully—have a better understanding of how A/B testing works, what it can do for you, and what mistakes to avoid, you should be better equipped to use such a powerful tool.
If you want to learn more about tools that make A/B testing easy, I recommend checking out my in-depth articles on Unbounce and ABtesting.ai.
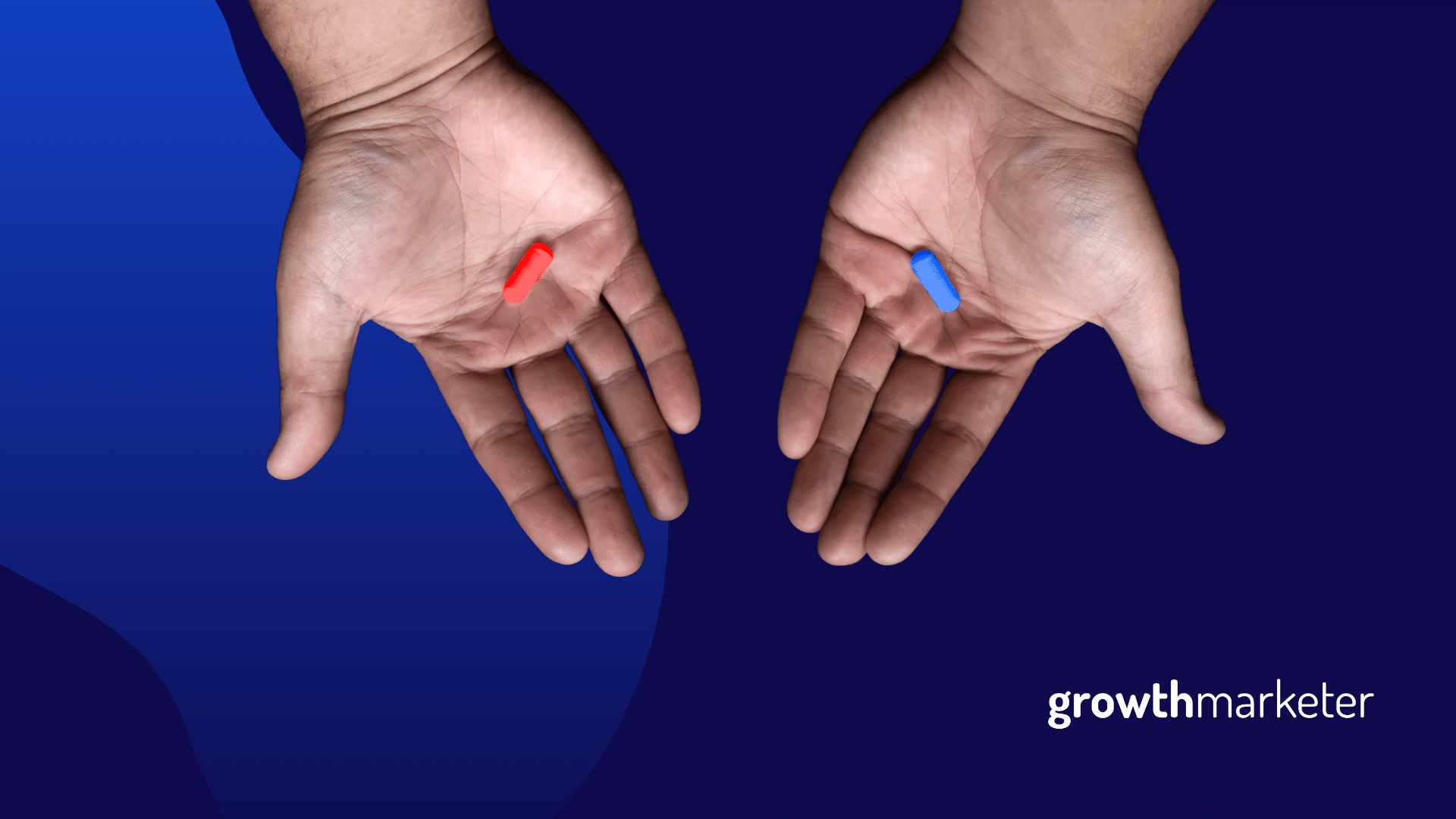